Share this
Building Credit Scores For Developing World’s Unbanked
by Ronen Shnidman on Feb 14, 2016
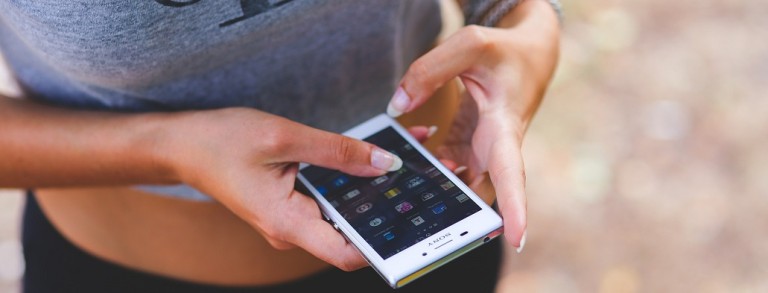
The financial services industry is spending a lot of effort to find technological solutions to provide credit to the 2 billion people, half the global working age population, who are unbanked. Little do they realize that all the technology they need is out there and already at their fingertips. All the credit agencies need to do is bring the existing technology together into one, effective solution.
The biggest problem facing the unbanked across the globe is the lack of formal records that can be used to create traditional credit scores. This means that even in most of the 145 countries surveyed by the World Bank affiliated Global Credit Bureau that have either privately run credit agencies or publicly run credit registries a large portion of the population is not covered (see map).
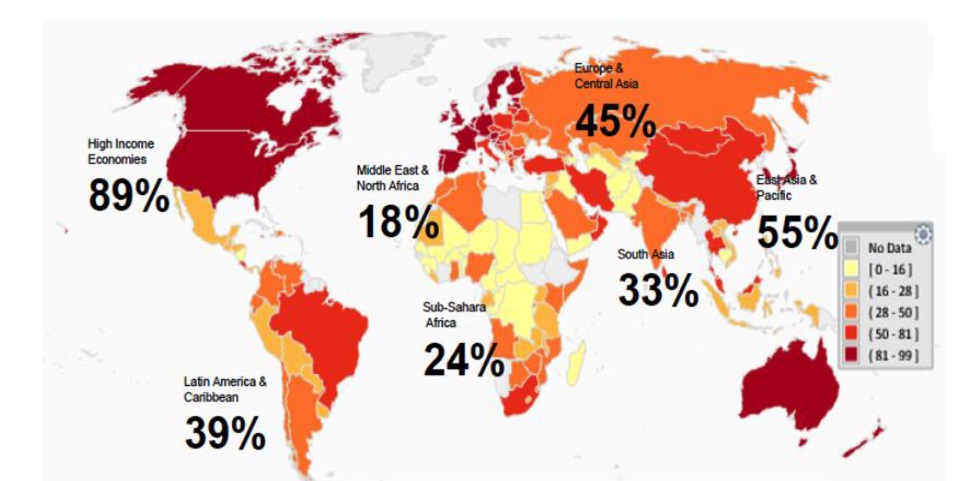
Mobile data: the savior of the unbanked
A simple solution is probably staring you in the face as you read: the mobile phone. The rapid spread of mobile phones mean that pretty soon most of the world will be making payments to their local telecom or cellphone service provider.
Ericsson’s Mobility Report in 2014 predicted that 90 percent of people around the globe above the age of six will have a mobile phone by 2020. Cisco more conservatively estimates that 70 percent of people around the globe, in absolute terms, 5.4 billion people, will have mobile phones by 2020.
This means that while half the world may lack formal credit records, almost all of them will have a history of financial and behavioral interactions with a cellular service provider by 2020. Excitingly, the high growth areas for cellphone use are also some of most interesting for areas for microfinance, alternative credit scoring and P2P lending, such as China, India and Sub-Saharan Africa.
The key is marrying personally identifiable information to behavioral data provided by mobile phone use.
Predicting credit risk with mobile behavioral data
Brown University economist Daniel Björkegren and Entrepreneurial Finance Lab CTO Darrell Grissen have shown in a Caribbean field study that prepaid cellphone usage and payment data can be effective in assessing finance risk for the un- and underbanked. Their study used anonymized linked data from a cellphone service provider and a microcredit cellphone and microcredit data found that a bank could reduce defaults by 41 percent while still accepting 75 percent of the underbanked individuals using cellphone data.
Björkegren and Grissen measured behavioral variables like whether individuals maintained a reserve on their pre-paid plans for emergency use and found them to have predictive power in determining default risk. To create credit profiles based on this information would require cooperation with telecoms. If telecoms chose to only provide anonymized data, credit agencies could skirt this problem by using an identity resolution service like Pipl to determine the identity of the owner of the phone number.
How to deal with address-free communication
A significant percentage of the population in low and middle income countries live in legally unrecognized townships and shantytowns that lack formal addresses. A lack of a legally registered residence may make it difficult to contact individuals via registered mail. But identity resolution technology provided by Pipl makes it possible to connect an individual’s phone number to their professional and personal emails and social media accounts. In some developing countries, as much as half the total population already have social media profiles, such as China. This makes it possible to cost-effectively maintain contact and legally recognized communication with individuals who lack an up to date home address.
Social risk scoring
Both mobile and social media data can be also be used for alternative risk scoring independent of financial data. For example, Björkegren and Grissen in their study measured how frequently participants’ calls were returned to measure the strength of their social ties. Something similar could also be done with social media accounts to measure the strength of an individual’s local connections and reputation when more traditional measures are lacking.
The idea of using social media for this purpose may sound new and a bit radical but it’s based on a very old standard for the setting of bail in common law. In such circumstances, it is very common for a judge to reduce or increase bail based on the strength of the person’s community ties. Where the person in question has few ties to the community he is presumed to be a higher flight risk and must pay a higher bail to mitigate that risk.
It would be pretty straightforward to apply that traditional concept of social risk to social media with a little though and some pre-implementation trials. Some obvious variables worth exploring for their ability to predict default risk include:
- Social profile account age – The older the better
- Number of connections/friends – Both very low and very large numbers could indicate higher risk
- Scores of friends on social media – Birds of a feather flock together and peer pressure may make someone more or less likely to misuse credit
- User engagement stats – People who are active in their online communities are probably more afraid of the damage to their reputation that could be caused by a default. Individuals who do not participate in larger communities face not as deterred by damage to their reputations.
The basic technology already exists to bring many of the unbanked around the world into the global financial system. What is lacking in many countries is a credit rating provider willing to innovate. As the middle class in developing countries grows, they will be looking for more credit options. The advantage will go to the credit agencies that move early into incorporating new data to include the masses of new consumers and small-scale entrepreneurs who will be looking for credit.
Original blog post written by former Pipl Technology Evangelist Ronen Shnidman. Ronen is now Managing Editor @ about-fraud.com