Share this
Seriously? This is Why You Can’t Prevent Fraud Without Data
by Admin on Nov 17, 2015
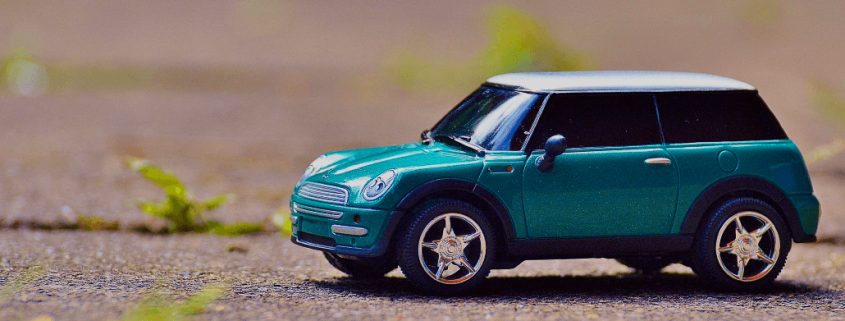
Here is a great example of online fraud.
Recently, my mother noticed some odd purchases on her debit card statement from companies she didn’t recognize. I’ve heard of these companies, and you probably have too, but for a very low-tech (“how do you work this iPhone?”) woman in her 60’s companies like Uber and Ali Express are complete mysteries.
My mom was obviously a victim of card not present (CNP) or online fraud, which reached over a thousand dollars. This was pretty worrying for my mom, but I was amused because it became a great object lesson about the failures of major fraud prevention platforms that I am sharing with you now.
In the two months since a fraudster took hold of my mother’s debit card details, they spent over a thousand dollars on numerous online services, Uber rides and orders for all sorts of other stuff.
Below, I’ll go into detail of how the online merchants, the debit card company and my mother’s bank each failed to catch this fraud. In addition, we’ll see how easy it should have been to spot the red flags if they only had the right data about my mom.
How the fraud detection platforms failed
There are 3 basic components to fraud detection platforms, particularly those that detect CNP fraud: behavioral profiling, velocity monitoring and customer profiling.
Behavior profiling checks transactions against past usage of a card or the average verified customer’s behavior to see if everything follows a regular pattern of behavior (items purchased, IP address, device fingerprint, etc.). Considering my mom only uses this card for groceries and pet supplies, the debit card’s behavior profiling failed big time here.
Velocity monitoring checks to see if a card is being used at an abnormal rate. This was another big fail here since at one point my mom’s card was used for Uber rides 11 times in one day! This kind of usage should have been flagged for fraud but Uber, the debit card company and my mom’s bank all ignored this.
Cardholder profiling means building a profile of a person from their real-life data, like age, gender, address, social profiles, etc. The fraud platforms used by all the companies involved scored another 0 since the purchases made with my mother’s credit card information did not match any purchase you’d expect a person with her profile to make.
What they should have looked at
Checking the velocity and behavior of the purchase seem pretty obvious but cardholder profiling is key to stopping fraud. Why? Because it’s a lot harder to keep fraudulent purchases in-line with a person’s profile than it is to fake behavior and purchase velocity.
Here is how the bank, debit card and merchants could have avoided losing hundreds of dollars each on the CNP fraud committed against my mother if they had good profile data:
AliExpress – poor address verification
Did AliExpress check this at all? What was the shipping address for all of the items ordered? Did it match my mom’s current or historical addresses? It didn’t, obviously.
With a good people data service (like Pipl’s for instance) not only would AliExpress see that items are being shipped to an address totally unrelated to my mom’s but also unrelated to anyone associated with l her.
Uber – no address verification
If Uber had any data about my mom, they would have realized that the pick-up and drop-off locations had nothing to do with my mom’s address or her associates’ addresses.
Uber – no social profile data
My mom is very low-tech. She’s a woman in her 60’s with no online presence at all – no Facebook, Twitter or Pinterest profile, no random web mentions or posts – nothing. Uber is a fairly high tech service – shouldn’t my mom’s lack of an online presence have raised some red flags? What’s the likelihood that someone without a Facebook profile would be an Uber user?
Not only that, but Uber is used mainly by young people in urban locations, my mom doesn’t fit this profile at all. The fraud score should’ve triggered all sorts of alerts when she suddenly used Uber’s service 11 times in one day.
AliExpress, Uber, the bank & debit card company – no email or phone verification
CNP purchases are made online or through a mobile phone. These kinds of purchases require entering either an email address or phone number or both.
The fraudster had to use my mom’s name and billing address to make the purchases. This is information could have easily been linked back to my mom’s email address and phone via a 3rd party API, like Pipl’s. Once the merchant, the debit card company or the bank had this info, they could have checked who owned the email or phone number – and discovered it was a person who is not my mother.
The next step would be to email or call my mom using her billing info or data found via a people data service to verify the purchase. They didn’t, of course.
As you can see, there are so many profile-based red flags that should have at a minimum pushed the fraud score higher. The contextless fraud prevention by the bank, the various merchants, and debit card company are to blame here.
How you should be using a person’s digital footprint to prevent CNP fraud
There are some basics that every fraud prevention platform should include when calculating fraud scores. Again, using some sort of people data API that returns a person’s online and offline data should be used in fraud detection to provide enough input to develop a score.
Address
Compare the billing and shipping addresses to the person’s actual address or historical addresses. If an address is different, especially the shipping address, this should increase the fraud score.
Is the email used for the purchase or by the user account linked to the person in the billing information?
If you run an email address lookup and the person returned is not the person in the billing info, the transaction is likely fraudulent. If no information is returned when looking up the email address, then the fraud score should increase somewhat.
Age
Does the person’s age make sense when looking at the product or service purchased? If not, it’s a red flag.
Phone
This is very similar to email addresses. The phone number used in the purchase should match the person in the billing info. Keep in mind that a phone number is not unique since several people can use the same number.
Associates
It’s important to have a good idea of who is connected to the purchaser. Why? It’s a smart way to fight chargebacks. Imagine someone is claiming purchases are fraudulent but the shipping address belongs to an associate. They are likely receiving the goods without having to pay for them – a common form of fraud.
Social profiles/digital footprint
You need to ask yourself two questions when it comes to a person’s digital footprint:
- If there is no digital footprint, does that make sense? In my mom’s case it did since people her age are a lot less likely to be active online.
- Does the digital footprint look authentic? It’s hard to fake a digital footprint that includes many different online sources, pages, directories, social networks, associates, etc.
Historical data
One of the most undervalued types of data are archived and historical data. A real person leaves a trail of connected data throughout his or her life.
- Address – does this person have multiple addresses? As you get older, you start to build a history of addresses.
- Social profiles – how old is the person’s social profile? If you find a person has multiple social profiles, you can feel a bit more confident they’re authentic, especially if the profiles weren’t created on the same date or time period and they’ve been in use for a while.
- Email address – email addresses aren’t changed all that often, so the older an email address is, the less likely it was created for fraudulent purposes.
Job
This is a simple one, does the person’s job make him or her more or less likely to buy a specific item or service. A cab driver is very unlikely to use Uber and a cashier might be less likely to make a very expensive purchase online.
So, how did the fraudster get his hands on my mom’s card details?
It’s hard to tell. My mom doesn’t make any online purchases and rarely uses the card in question.
One thing I do know is that CNP fraud is a vibrant industry, expected to cost e-commerce vendors over $19 billion and $3.10 in losses for ever $1 in fraudulent purchases. As a result, it definitely pays for fraud prevention platforms and merchants to use every piece of data at their disposal to prevent fraud.